Pemodelan Topik pada Ulasan Google Maps Candi Borobudur Menggunakan Latent Dirchlet Allocation

(1) Universitas Kristen Immanuel 

(2) Universitas Kristen Immanuel 

(3) Universitas Kristen Immanuel 

(4) Universitas Kristen Immanuel 


DOI : https://doi.org/10.24036/voteteknika.v12i3.129028
Full Text:
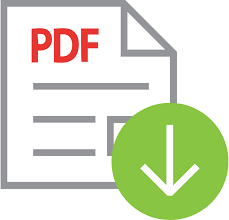
Abstract
Penelitian ini bertujuan untuk menggali wawasan yang mendalam dari ulasan Google Maps wisatawan tentang Candi Borobudur menggunakan metode Pemodelan Topik Latent Dirichlet Allocation(LDA). Candi Borobudur, salah satu situs warisan dunia yang terkenal di Indonesia, menerima banyak ulasan dari wisatawan yang berbeda-beda. Namun, untuk mengidenfikasi tren, preferensi, dan aspek yang paling penting bagi pengunjung, diperlukan analisis yang lebih dalam. Dengan menggunakan pendekatan LDA, mengelompokkan ulasan-ulasan ini kedalam topik-topik latensial yang memungkinkan untuk mengeksplorasi pola-pola umum dan perbedaan-perbedaan dalam persepsi dan pengalaman pengunjung terhadap Candi borobudur. Proses pemodelan topik ini mencakup beberapa langkah, dimulai dari persiapan data, pra-pemrosesan data, wordcloud, topic coherence serta pemodelan topik. Adapun nilai koherensi paling baik diperoleh dalam model Latent dirichlet allocation (LDA) adalah topik terbaik dengan jumlah 4 topik. Sedangkan dari hasil analisis pemodelan topik pada metode Latent dirichlet allocation (LDA) pada Kata "candi" (0.050883695), "borobudur" (0.02406518), "tiket" (0.018967053), dan "masuk" (0.018507887), menyoroti fokus pada aspek kuil, aksesibilitas, dan biaya masuk. Kata "jalan" (0.012353696) dan "parkir" (0.011362941) menunjukkan perhatian terhadap infrastruktur, sementara "area" (0.01039175) mengacu pada lingkungan sekitar candi. Istilah "rb" (0.009597249) dan "harga" (0.0092093265) merujuk pada biaya dalam rupiah, dan "beli" (0.008586656) terkait dengan pembelian tiket. Hasil ini memberikan wawasan tentang fokus utama dalam pembahasan Borobudur, mencakup kuil, aksesibilitas, infrastruktur, dan biaya.
Kata kunci: Google Maps, Pemodelan Topik, Latent Dirichlet Alloation(LDA), Nilai Koherensi
This research aims to extract deep insights from tourists' Google Maps reviews of Borobudur Temple using the Latent Dirichlet Allocation (LDA) Topic Modeling method. Borobudur Temple, one of the famous world heritage sites in Indonesia, receives many reviews from different tourists. However, to identify trends, preferences, and aspects that are most important to visitors, a deeper analysis is required. Using the LDA approach, categorizing these reviews into latent topics makes it possible to explore common patterns and differences in visitors' perceptions and experiences of Borobudur Temple. This topic modeling process includes several steps, starting from data preparation, data pre-processing, wordcloud, topic coherence and topic modeling. The best coherence value obtained in the Latent dirichlet allocation (LDA) model is the best topic with a total of 4 topics.. While based on the results of the analysis of topic modeling with the Latent dirichlet allocation (LDA) method on Borobudur reviews get 4 topic models The best coherence value obtained in the Latent dirichlet allocation (LDA) model is the best topic with a total of 4 topics. While from the results of the topic modeling analysis on the Latent dirichlet allocation (LDA) method on the words “temple” (0.050883695), “borobudur” (0.02406518), “ticket” (0.018967053), and “entrance” (0.018507887), highlighting the focus on aspects of the temple, accessibility, and entrance fees. The words “road” (0.012353696) and “parking” (0.011362941) indicate attention to infrastructure, while “area” (0.01039175) refers to the temple's surroundings. The terms “rb” (0.009597249) and “harga” (0.0092093265) refer to costs in rupiah, and “beli” (0.008586656) is related to ticket purchases. These results provide insight into the main focus of the Borobudur discussion, covering temples, accessibility, infrastructure, and costs.
Keywords: Google maps, topic modelling, Latent Dirichlet Alloation(LDA), Coherence Score
References
Y. Afrillia, L. Rosnita, and D. Siska, “Analisis Sentimen Ciutan Twitter Terkait Penerapan Permendikbudristek Nomor 30 Tahun 2021 Menggunakan TextBlob dan Support Vector Machine,” G-Tech: Jurnal Teknologi Terapan, vol. 6, no. 2, pp. 387–394, Oct. 2022, doi: 10.33379/gtech.v6i2.1778.
Y. Matira and I. Setiawan, ‘Pemodelan Topik pada Judul Berita Online Detikcom Menggunakan Latent Dirichlet Allocation’, Estimasi: Journal of Statistics and Its Application, vol. 4, no. 1, pp. 2721–379, 2023, doi: 10.20956/ejsa.vi.24843.
R. Abdillah, E. Haerani, and R. M. Candra, “Analisis Sentimen Ulasan Aplikasi Wetv Untuk Peningkatan Layanan Menggunakan Metode Support Vector Machine,” Journal of Information System Research (JOSH), vol. 4, no. 3, pp. 865–873, Apr. 2023, doi: 10.47065/josh.v4i3.3353.
W. A. Nawang Sari and H. Dwi Purnomo, ‘Topic Modeling Using the Latent Dirichlet Allocation Method on Wikipedia Pandemic Covid-19 Data in Indonesia’, Jurnal Teknik Informatika (Jutif), vol. 3, no. 5, pp. 1223–1230, 2022, doi: 10.20884/1.jutif.2022.3.5.321.
Sari and Wilujeng Ayu Nawang, “Pemodelan Topik Menggunakan Metode Latent Dirichlet Allocation Pada Data Wikipedia Pandemic Covid- 19 di Indonesia”, https://repository.uksw.edu/handle/123456789/26 150.
M. Taufiq Anwar, D. Riandhita Arief Permana, “Analisis Sentimen Masyarakat Indonesia Terhadap Produk Kendaraan Listrik Menggunakan VADER,” Jurnal Teknik Informatika dan Sistem Informasi, vol. 10, no. 1, pp. 783–792, Mar. 2023,
D. Aryanti, “Analisis Sentimen Ibukota Negara Baru Menggunakan Metode Naïve Bayes Classifier,” Journal of Information System Research (JOSH), vol. 3, no. 4, pp. 524–531, Jul. 2022, doi: 10.47065/josh.v3i4.1944.
L. O. Sihombing, H. Hannie, and B. A. Dermawan, “Sentimen Analisis Customer Review Produk Shopee Indonesia Menggunakan Algortima Naïve Bayes Classifier,” Edumatic: Jurnal Pendidikan Informatika, vol. 5, no. 2, pp. 233–242, Dec. 2021, doi: 10.29408/edumatic.v5i2.4089.
Rianita Giovanni Katryn, ‘Text Preprocessing: Tahap Awal dalam Natural Language Processing (NLP)’, https://medium.com/mandiri- engineering/text-preprocessing-tahap-awal-dalam- natural-language-processing-nlp-bc5fbb6606a.
R. Rinandyaswara, Y. A. Sari, and M. T. Furqon, ‘PEMBENTUKAN DAFTAR STOPWORD MENGGUNAKAN TERM BASED RANDOM SAMPLING PADA ANALISIS SENTIMEN DENGAN METODE NAÏVE BAYES (STUDI KASUS: KULIAH DARING DI MASA PANDEMI)’, vol. 9, no. 4, 2022, doi: 10.25126/jtiik.202294707.
N. Hendrastuty, A. Rahman Isnain, and A. Yanti Rahmadhani, “Analisis Sentimen Masyarakat Terhadap Program Kartu Prakerja Pada Twitter Dengan Metode Support Vector Machine,” Jurnal Pengembangan IT (JPIT), vol. 6, no. 3, Jul. 2021.
D. Normawati and S. A. Prayogi, “Implementasi Naïve Bayes Classifier Dan Confusion Matrix Pada Analisis Sentimen Berbasis Teks Pada Twitter,” Jurnal Sains Komputer & Informatika (J-SAKTI), vol. 5, no. 2, pp. 697–711, Sep. 2021.
Nurdin, K. F., Sutanto, T. E., & Santoso, A. (2023). Analisa Pemodelan Topik Berita Daring Menggunakan Semi-Supervised dan Fully Unsupervised Latent Dirichlet Allocation. Jurnal Pendidikan Tambusai, 7(2), 8043–8053. https://www.palupos.com
W. Sejati, A. Singh Bist, and A. Tambunan, “Pengembangan Analisis Sentimen dalam Rekayasa Software Engineering menggunakan tinjauan literatur sistematis,” Jurnal Manajemen Pendidikan dan Teknologi Informasi , vol. 2, no. 1, pp. 95–103, Sep. 2023
A. Dwiki, A. Putra, and S. Juanita, “Analisis Sentimen Pada Ulasan Pengguna Aplikasi Bibit Dan Bareksa Dengan Algoritma KNN,” Jurnal Teknik Informatika dan Sistem Informasi, vol. 8, no. 2, pp. 636–646, Jun. 2021.
Article Metrics


Refbacks
- There are currently no refbacks.

This work is licensed under a Creative Commons Attribution-ShareAlike 4.0 International License.