Klasifikasi Penyakit Stroke Menggunakan Metode Naïve Bayes Classification dan Chi-Square Feature Selection

(1) Universitas Jenderal Achmad Yani 

(2) Universitas Jenderal Achmad Yani 

(3) Universitas Jenderal Achmad Yani 


DOI : https://doi.org/10.24036/voteteknika.v12i3.129022
Full Text:
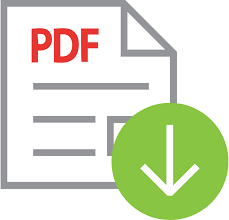
Abstract
Penyakit stroke merupakan suatu penyakit yang dapat memutuskan suplai darah menuju otak. Menurut World Health Organization (WHO), stroke merupakan salah satu penyebab kematian tertinggi di dunia. Penelitian ini bertujuan untuk menggunakan teknik klasifikasi untuk mendeteksi tingkat resiko terkena penyakit stroke. Klasifikasi merupakan teknik yang bertujuan untuk memperkirakan kelas dari suatu objek yang kelasnya masih tidak diketahui. Penelitian ini mengkombinasikan salah satu metode dari klasifikasi yaitu Naive Bayes dengan salah satu metode seleksi fitur yaitu Chi-Square untuk meningkatkan akurasi dari klasifikasi Naive Bayes. Hasil penelitian ini menunjukkan bahwa seleksi fitur Chi-Square terbukti dapat meningkatkan akurasi pada klasifikasi Naive Bayes dalam klasifikasi penyakit stroke dengan pembagian data latih dan uji yaitu 75:25. Hasil akurasi meningkat dari 73,55% sebelum menggunakan metode seleksi fitur Chi-Square menjadi 74,94% setelah menggunakan metode seleksi fitur Chi-Square. Penelitian ini diharapkan dapat membuka wawasan baru terkait metode seleksi fitur Chi-Square dalam meningkatkan kinerja dari suatu metode klasifikasi khususnya dalam mendeteksi risiko penyakit stroke sebagai tindakan pencegahan dan penanganan risiko penyakit stroke.
Kata kunci: Chi-Square, klasifikasi,, Naive Bayes, Stroke.
Stroke is a disease that can cut off the blood supply to the brain. According to the World Health Organization (WHO), stroke is one of the highest causes of death in the world. This study aims to use classification techniques to detect the risk level of stroke. Classification is a technique that aims to estimate the class of an object whose class is unknown. This research combines one of the classification methods, Naive Bayes, with one of the feature selection methods, Chi-Square, to improve the accuracy of Naive Bayes classification. The results of this study show that Chi-Square feature selection is proven to improve the accuracy of Naive Bayes classification on stroke disease classification with a division of training data and test data of 75:25. The accuracy results increased from 73.55% before using the Chi-Square feature selection method to 74.94% after using the Chi-Square feature selection method. This research is expected to open new insights related to the Chi-Square feature selection method in improving the performance of a classification method, especially in detecting the risk of stroke disease as a preventive measure and handling the risk of stroke disease.
Keywords: Chi-Square, Classification, Naive Bayes, Stroke.
References
A. Puspitawuri, E. Santoso, and C. Dewi, “Diagnosis Tingkat Risiko Penyakit Stroke Menggunakan Metode K-Nearest Neighbor dan Naïve Bayes,” J. Pengemb. Teknol. Inf. dan Ilmu Komput., vol. 3, no. 4, pp. 3319–3324, 2019.
M. S. Phipps and C. A. Cronin, “Management of acute ischemic stroke,” BMJ, vol. 368, 2020, doi: 10.1136/bmj.l6983.
A. Byna and M. Basit, “Penerapan Metode Adaboost Untuk Mengoptimasi Prediksi Penyakit Stroke Dengan Algoritma Naïve Bayes,” vol. 09, no. November, pp. 407–411, 2020.
V. Adelina, D. E. Ratnawati, and M. A. Fauzi, “Klasifikasi Tingkat Risiko Penyakit Stroke Menggunakan Metode GA-Fuzzy Klasifikasi Tingkat Risiko Penyakit Stroke Menggunakan Metode GA- Fuzzy Tsukamoto,” J. Pengemb. Teknol. Inf. dan Ilmu Komput. Univ. Brawijaya, vol. 2, no. September, pp. 3015–3021, 2018.
D. Ulfatul, M. Rachmad, H. Oktavianto, and M. Rahman, “Perbandingan Metode K-Nearest Neighbor Dan Gaussian Naive Bayes Untuk Klasifikasi Penyakit Stroke Comparison Of K-Nearest Neighbor And Gaussian Naive Bayes Methods For Stroke Disease Classification Jurnal Smart Teknologi bidang pengenalan pola , kategori,” J. Smart Teknol., vol. 3, no. 4, pp. 405–412, 2022.
I. Budiman and R. Ramadina, “Penerapan Fungsi Data Mining Klasifikasi untuk Prediksi Masa Studi Mahasiswa Tepat Waktu pada Sistem Informasi Akademik Perguruan Tinggi,” Ijccs, vol. x, No.x, no. 1, pp. 1–5, 2015.
H. Annur, “Klasifikasi Masyarakat Miskin Menggunakan Metode Naive Bayes,” Ilk. J. Ilm., vol. 10, no. 2, pp. 160–165, 2018, doi: 10.33096/ilkom.v10i2.303.160-165.
D. Sartika and D. I. Sensuse, “Perbandingan Algoritma Klasifikasi Naive Bayes, Nearest Neighbour, dan Decision Tree pada Studi Kasus Pengambilan Keputusan Pemilihan Pola Pakaian,” Jatisi, vol. 1, no. 2, pp. 151–161, 2017.
H. Apriyani and K. Kurniati, “Perbandingan Metode Naïve Bayes Dan Support Vector Machine Dalam Klasifikasi Penyakit Diabetes Melitus,” J. Inf. Technol. Ampera, vol. 1, no. 3, pp. 133–143, 2020, doi: 10.51519/journalita.volume1.isssue3.year2020.page133-143.
S. Paudel, P. W. C. Prasad, A. Alsadoon, M. R. Islam, and A. Elchouemi, “Feature selection approach for twitter sentiment analysis and text classification based on chi-square and naïve bayes,” Adv. Intell. Syst. Comput., vol. 842, pp. 281–298, 2019, doi: 10.1007/978-3-319-98776-7_30.
X. Deng, Y. Li, J. Weng, and J. Zhang, “Feature selection for text classification: A review,” Multimed. Tools Appl., vol. 78, no. 3, pp. 3797–3816, 2019, doi: 10.1007/s11042-018-6083-5.
S. Thaseen and A. Kumar, “Intrusion detection model using fusion of chi-square feature selection and multi class SVM,” J. King Saud Univ. - Comput. Inf. Sci., vol. 29, no. 4, pp. 462–472, 2017, doi: 10.1016/j.jksuci.2015.12.004.
S. Hadijah Hasanah, “Application of Machine Learning for Heart Disease Classification Using Naive Bayes,” J. Mat. MANTIK, vol. 8, no. 1, pp. 68–77, 2022, doi: 10.15642/mantik.2022.8.1.68-77.
Y. N. Paramitha, A. Nuryaman, A. Faisol, E. Setiawan, and D. E. Nurvazly, “Klasifikasi Penyakit Stroke Menggunakan Metode Naïve Bayes,” J. Siger Mat., vol. 04, no. 01, pp. 11–16, 2023.
C. Chairunnisa, I. Ernawati, and M. M. Santoni, “Klasifikasi Sentimen Ulasan Pengguna Aplikasi PeduliLindungi di Google Play Menggunakan Algoritma Support Vector Machine dengan Seleksi Fitur Chi-Square,” Inform. J. Ilmu Komput., vol. 18, no. 1, p. 69, 2022, doi: 10.52958/iftk.v17i4.4594.
V. Zuliana, I. Maulana, and U. S. Karawang, “Analisis Sentimen Program Migrasi Tv Digital Menggunakan,” no. 2, pp. 90–95, 2022.
H. M. Lumbantobing, R. A. Marcellino, and I. C. Bu’ulolo, “Penerapan Metode Feature Selection pada Algoritma Naïve Bayes dalam Kasus Keyword Extraction,” Citee, pp. 117–123, 2020.
Article Metrics


Refbacks
- There are currently no refbacks.

This work is licensed under a Creative Commons Attribution-ShareAlike 4.0 International License.