Klasifikasi Analisis Tekstur Citra Radiografi Panoramik Gigi Untuk Deteksi Osteoporosis Menggunakan Metode K-Nearest Neighbor (KNN)

(1) Universitas Negeri Padang 

(2) Universitas Negeri Padang 

(3) Universitas Negeri Padang 


DOI : https://doi.org/10.24036/voteteknika.v11i3.124871
Full Text:
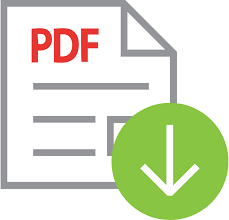
Abstract
Osteoporosis merupakan salah satu penyakit yang berkaitan dengan proses penuaan (degeneratif) yang diindikasikan dengan terjadinya penurunan kerapatan yang cepat dan penipisan jaringan tulang sehingga terjadi penurunan kekuatan mekanik tulang dalam mendukung aktivitas sehari-hari. Teknik yang banyak dikembangkan untuk pengukuran yang berkaitan dengan massa tulang, serta dianggap sebagai gold standard adalah Dual Energi X-ray Absorptiometry (DXA). Beberapa penelitian sebelumnya memberikan peluang pemanfaatan citra radiografi panoramik gigi untuk analisis kerapatan trabekula tulang mandibula. Hasil ini digunakan sebagai sarana dekteksi dini kondisi Bone Mineral Density (BMD). Penelitian ini bertujuan melakukan klasifikasi terhadap hasil analisis tekstur menggunakan prinsip Grey Level Co-occurence Matrix (GLCM) pada citra panoramik gigi. Dari metode GLCM diperoleh ekstraksi fitur yang selanjutnya dijadikan input bagi K-Nearest Neighbor (KNN) untuk melakukan klasifikasi. Uji coba dilakukan menggunakan data BMD vertebra lumbar dan citra panoramik gigi 23 sampel wanita berusia antara 52 – 73 tahun yang telah memasuki masa postmenopause. Hasil klasifikasi kelas normal dan osteoporosis menggunakan KNN (9 data latih dan 14 data uji) memberikan pengenalan paling baik dengan akurasi 78,57%, sensitivitas (tingkat benar positif) 100% dan spesifisitas (tingkat benar negatif) 66,67%. Pengenalan paling baik didapatkan menggunakan fitur contrast, energy, dan homogeneity dan kombinasi ketiganya sebagai input bagi klasifikasi KNN.
Kata kunci : Osteoporosis, citra radiografi panoramik, analisis tekstur, Grey Level Co-occurrence Matrix (GLCM), K-Nearest Neighbor (KNN).
Osteoporosis is a degenerative disease which is characterized by a rapid decrease in bone density resulting in a decrease in the mechanical strength of the bones to support daily activities. The gold standard for bone mass measurement is Dual Energy X-ray Absorptiometry (DXA). Several previous studies provided the opportunity to utilize dental panoramic radiographic images for analysis of mandibular trabecular bone density. These results are used as an early detection tool for Bone Mineral Density (BMD) conditions. This research tries to use the feature extraction results of texture analysis using Grey Level Co-occurrence Matrix (GLCM) on dental panoramic images as input for K-Nearest Neighbor (KNN) to carry out classification. The trial was conducted using lumbar vertebral BMD data and dental panoramic images from 23 samples of women aged between 52-73 years who had entered the postmenopausal period. Classification results of normal and osteoporosis using KNN (9 training data and 14 test data) gave the best recognition with an accuracy of 78.57%, a sensitivity of 100% and a specificity of 66.67%. The best recognition is obtained by using contrast, energy, and homogeneity features and their combination as input for KNN classification.
Keywords: Osteoporosis, dental panoramic radiograph, texture analysis, Grey Level Co-occurrence Matrix (GLCM), K-Nearest Neighbor (KNN) .
References
WHO Technical Report Series, Prevention and management of osteoporosis : report of a WHO Scientific Group., vol. 921. World Health Organization, 2003.
Suprijanto, E. Juliastuti, Y. Diputra, and M. Mayantasari, Dental Panoramic Image Analysis on Mandibular Bone for Osteoporosis Early Detection, Proceedings 3rd International Conference on ICA, Bali, Indonesia, pp. 138-143, August, 2013.
R. Abdalla-Aslan, T. Yeshua, D. Kabla, I. Leichter, and C. Nadler, “An Artificial Intelligence System using Machine-Learning for Automatic Detection and Classification of Dental Restorations in Panoramic Radiography,” Oral Surg Oral Med Oral Pathol Oral Radiol, vol. 130, no. 5, pp. 593–602, Nov. 2020, doi: 10.1016/j.oooo.2020.05.012.
W. R. Wulandari, S. A. Wibowo, and D. Fahmi Oscandar, “Klasifikasi Umur Berdasarkan Citra Dental Panoramic Radiograph Dengan Metode Statistical Analysis of Structural Information dan Adaboosts,” e-Proceedings of Engineering, vol. 6, No.2, pp. 4216-4222, Agustus, 2019.
K. Horner and H. Devlin, “The Relationships Between Two Indices of Mandibular Bone Quality and Bone Mineral Density Measured by Dual Energy X-Ray Absorptiometry,” Dentomaxillofacial Radiology, vol.27, pp. 17-21, 1998.
P. D. Allen et al., “Detecting reduced bone mineral density from dental radiographs using statistical shape models,” IEEE Transactions on Information Technology in Biomedicine, vol. 11, no. 6, pp. 601–610, Nov. 2007, doi: 10.1109/TITB.2006.888704.
P. C. A. Watanabe, J. P. M. Issa, S. A. C. Monteiro, M. M. Iyomasa, S. C. H. Regalo, and S. Siessere, “Morphodigital Study of the Mandibular Trabecular Bone in Panoramic Radiographs,” International Journal of Morphology, vol.25, no.4, pp.875-880, 2007.
A. Z. Arifin, A. Asano, A. Taguchi, T. Nakamoto, M. Ohtsuka, and K. Tanimoto, “Computer-aided system for measuring the mandibular cortical width on panoramic radiographs in osteoporosis diagnosis,” in Medical Imaging 2005: Image Processing, SPIE, Apr. 2005, pp. 813–821. doi: 10.1117/12.594458.
C. Wijaya, H. Irsyad, and W. Widhiarso, “Klasifikasi Pneumonia Menggunakan Metode K-Nearest Neighbor Dengan Ekstraksi GLCM,” Jurnal Algoritme, Vol.1, No.1, pp. 33-44, Oktober, 2020.
R. Yunus, U. Ulfa, and M. D. Safitri, “Application of the K-Nearest Neighbors (K-NN) Algorithm for Classification of Heart Failure,” Journal of Applied Inteligence System, Vol.6, No.1, pp. 1-9, 2021.
J. Elektronik, I. K. Udayana, D. Reness, N. A1, and B. Jimbaran, “Klasifikasi Citra Rontgen Gigi Berdasarkan Analisis Tekstur Untuk Diagnosis Karies Gigi Manusia Menggunakan Metode SVM,” Jurnal Elektronik Ilmu Komputer Udayana, vol. 9, no. 1, pp. 141–150, 2020.
N. Kadek Ayu Wirdiani, P. Hridayami, N. Putu Ayu Widiari, K. Diva Rismawan, P. Bagus Candradinatha, and I. Putu Deva Jayantha, “Face Identification Based on K-Nearest Neighbor,” Scientific Journal of Informatics, vol. 6, no. 2, pp. 2407–7658, 2019, [Online]. Available: http://journal.unnes.ac.id/nju/index.php/sji
R. M. Haralick, Shanmugam K, and Dinstein Its’hak, “Textural Features for Image Classification,” IEEE Trans Syst Man Cybern, vol. SMC-3, No.6, no. November 1973, pp. 610–621, 1973.
D. Gadkari, “Image Quality Analysis Using GLCM,” MSc Thesis, University of Central Florida, Orlando, Florida,2000.
S. Martha, W. Andani, and S. W. Rizki, “Perbandingan Metode k-Nearest Neighbor, Regresi Logistik Biner, dan Pohon Klasifikasi pada Analisis Kelayakan Pemberian Kredit,” Euler : Jurnal Ilmiah Matematika, Sains dan Teknologi, vol. 10, no. 2, pp. 262–273, Nov. 2022, doi: 10.34312/euler.v10i2.16751.
Article Metrics


Refbacks
- There are currently no refbacks.

This work is licensed under a Creative Commons Attribution-ShareAlike 4.0 International License.